Instance segmentation based precise object detection in UAV Im-ages using Mask R-CNN
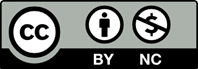
Object detection plays a vital role in remote-sensing datasets which trains the image or things and helps in classi-fying the images into their classes. Instance segmentation is the avant-garde technique used for object detection in Deep Learning. There are many instance segmentation models which can produce significant results. Object de-tection, segmentation, and RGB analysis in images taken from Unmanned Aerial Vehicles (UAV) are difficult with the desired level of performance. Instance segmentation is a powerful method that extracts each object and its location with the predicted label for pixels in the input image. In this paper, a study has been carried out on the implementation of Mask R-CNN for instance segmentation with different optimization algorithms to obtain a more accurate result for UAV images. The training has been carried out with Mask R-CNN for object detection using ResNet50 and ResNet101 as the backbone. After extensive experiments, it has been observed that the optimization algorithm plays a vital role in the overall computational process and can improve the accuracy level with a reduc-tion in the training/validation loss. The experiment has been conducted on publicly available UAV datasets. The paper further presents the results in terms of different performance parameters
- A Olgac & Bekir Karlik. (Feb. 2011). Performance Analysis of Various Activation Functions in Generalized MLP Architectures of Neural Networks. Artificial Intelligence and Expert Systems, 1, 111–122.
- Kaiming He et al. (2016). Deep Residual Learning for Image Recognition. IEEE Conference on Computer Vision and Pattern Recognition (CVPR), 770–778.
- Fengbao Yang, Yingjie Liu, & Peng Hu (2017). Small-Object Detection in UAV-Captured Images via Multi-branch Parallel Feature Pyramid Networks. IEEE Access, DOI: 10.1109/ACCESS.2020.3014910.
- K. He, G., Gkioxari, P. Dollár, & R. Girshick (2017). Mask R-CNN. IEEE International Conference on Computer Vision (ICCV), Venice, Italy, 2017, pp. 2980-2988, doi: 10.1109/ICCV.2017.322.
- Pathak, Ajeet, Pandey, Manjusha, & Rautaray, Siddharth. (2018). Application of Deep Learning for Object Detection. Procedia Computer Science, 132, 1706-1717. doi: 10.1016/j.procs.2018.05.144.
- Konstantin Eckle & Johannes Schmidt-Hieber (2019). A comparison of deep networks with ReLU activation function and linear spline-type methods. Neural Networks, 110, 232-242, 0893-6080.
- Renu Khandelwal (2019). Computer Vision: Instance Segmentation with Mask R-CNN.
- Padmalaya Nayak & Ravalisri Vasam (2019). Instance Segmentation on Real time Object Detection using Mask R-CNN. Engineering and Advanced Technology, 9(1).
- Dalal Al-Alimi, Yuxiang Shao, Ahamed Alalimi & Ahmed Abdu (2020). Mask R-CNN for Geospatial Object Detection. I.J. Information Technology and Computer Science, 2020, 5, 63-72.
- Sharma, Siddharth, Sharma, Simone, & Athaiya, Anidhya. (2020). Activation Functions in Neural Networks. International Journal of Engineering Applied Sciences and Technology, 04, 310-316.
- He K, Gkioxari G, Dollar P, & Girshick R (2020). Mask R-CNN. IEEE Trans Pattern Anal Mach Intell, 42(2): 386-397.
- Mahmoud, Amira, Mohamed, Sayed, El-Khoribi, Reda, & AbdelSalam, Hisham. (2020). Object Detection Using Adaptive Mask RCNN in Optical Remote Sensing Images. International Journal of Intelligent Engineering and Systems, 13, 65-76. doi: 10.22266/ijies2020.0229.07.
- R. Parhi and R. D. Nowak. (2020). The Role of Neural Network Activation Functions. IEEE Signal Processing Letters, 27, 1779–1783.
- R. Parhi & R. D. Nowak (2020). The Role of Neural Network Activation Functions. IEEE Signal Processing Letters, vol. 27, pp. 1779-1783.
- Zhang Yiqing, Jun Chu, Lu Leng, & Jun Miao (2020). Mask-Refined R-CNN: A Network for Refining Object Details in Instance Segmentation. Sensors, 20(4), 1010. https://doi.org/10.3390/s20041010.
- Chenfan Sun, Wei Zhan, Jinhiu She, & Yangyang Zhang (2020). Object Detection from the Video Taken by Drone via Convolutional Neural Networks. Mathematical Problems in Engineering, vol. 2020, Article ID 4013647.
- Jaikumar, Punitha, Vandaele, Remy, & Ojha, Varun. (2021). Transfer Learning, for Instance, Segmentation of Waste Bottles Using Mask R-CNN Algorithm. In: 10.1007/978-3-030-71187-0_13.
- Vaddi, Subrahmanyam, Kim, Dongyoun, Kumar, Chandan, Shad, Shafqat, & Jannesari, Ali. (2021). Efficient Object Detection Model for Real-time UAV Application. Computer and Information Science, 14(1), 45. doi: 10.5539/cis.v14n1p45.
- Cong Lin, Shijie Zhang, Shaodi You, Xiaoxiang Liu, & Zhiyu Zhu (2021). Real-time foreground object segmentation networks using long and short skip connections. Information Sciences, Volume 571, Pages 543-559, ISSN 0020-0255.
- Lucas Prado Osco, José Marcato Junior, Ana Paula Marques Ramos, Lúcio André de Castro Jorge, Sarah Narges Fatholahi, Jonathan de Andrade Silva, Edson Takashi Matsubara, Hemerson Pistori, Wesley Nunes Gonçalves, & Jonathan Li (2021). A review on deep learning in UAV remote sensing. International Journal of Applied Earth Observation and Geoinformation, Volume 102, 102456, ISSN 1569-8432.
- S. L. Ullo et al., (2021). A New Mask R-CNN-Based Method for Improved Landslide Detection. IEEE Journal of Selected Topics in Applied Earth Observations and Remote Sensing, vol. 14, pp. 3799-3810.
- Begum, S. R., Datta, S. Y. & Manoj, M. S. V. (2021). Mask R-CNN For Fire Detection. International Research Journal of Computer Science, VIII, 145-151.
- Chen, Leiyu, Shaobo Li, Qiang Bai, Jing Yang, Sanlong Jiang, & Yanming Miao. (2021). Review of Image Classification Algorithms Based on Con-volutional Neural Networks.Remote Sensing 13, no. 22: 4712. https://doi.org/10.3390/rs13224712.
- Muhammad Asif Saleem, Norhalina Senan, Fazli Wa-hid, Muhammad Aamir, Ali Samad, & Mukhtaj Khan (2022). Comparative Analysis of Recent Ar-chitecture of Convolutional Neural Network, Math-ematical Problems in Engineer-ing,9,https://doi.org/10.1155/2022/7313612.