Deep attention network with sentence-level classification-based sentiment analysis in Telugu considering linguistic feature
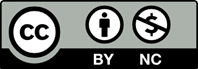
Sentiment analysis in conversations has gained increasing attention for the growing number of applications like human-robot interactions. Inaccurate emotion identification in existing sentiment analysis methods is due to a lack of concentration on explicit and implicit factors in sentiment detection. Hence a novel deep attention expression analysis technique has been introduced in which a deep attention network with parallel level fuzzy classifier identifies the nature of the words using sequential word N-gram functions by incorporating distributed semantics thereby the implicit and explicit nature of the sentence is identified and classified. Moreover, negations and tone in the sentence create a perplexity nature of sentiment analysis.To solve this problem,linguistic feature-based classification has been presented that utilizesa POS-based tagging in the attention layer and BOW to provide word embedding. Then, lemmatization and stemming process of words the root words are identified by maximum likeliness probability, resulting in the identification of new words with linguistic features. Furthermore, Naïve Bayes classifier and ensemble clustering with lambda function havebeen used to identify the negations and tone of the sentence. Thus, the results provided accurate detection of the positive, negative, or neutral sentiment of the sentence with a high accuracy of 96% and a precision of 97%.
- Badugu, & Srinivasu. (2020). Telugu Movie Review Sentiment Analysis Using Natural Language Processing Approach. Data Engineering and Communication Technology. Springer, Singapore, 685-695.
- Chiranjeevi, P., Teja Santosh, D., & Vishnuvardhan, B. (2019). Survey on Sentiment Analysis Methods for Reputation Evaluation. Cognitive Informatics and Soft Computing. Springer, Singapore, 53-66.
- Garapati, Abhinav, et al. (2019). SentiPhraseNet: An extended SentiWordNet approach for Telugu sentiment analysis. International Journal of Advance Research, Ideas and Innovations in Technology.
- Hasan, Mahmudul, Ishrak Islam, & Azharul Hasan, K. M. (2019). Sentiment Analysis Using Out of Core Learning. 2019 International Conference on Electrical, Computer and Communication Engineering (ECCE). IEEE.
- Jonnalagadda, Pravarsha, et al. (2019). A rule-based sentiment analysis in Telugu. International Journal of Advance Research, Ideas and Innovations in Technology.
- Joshi, & Aditya. (2019). Sentiment Analysis and Opinion Mining from Noisy Social Media Content. Diss. PhD Thesis, International Institute of Information Technology Hyderabad.
- Juhn, Young, & Hongfang Liu. (2020). Artificial intelligence approaches using natural language processing to advance EHR-based clinical research. Journal of Allergy and Clinical Immunology, 145(2), 463-469.
- Kujur, Fedric, & Saumya Singh. (2020). Visual communication and consumer-brand relationship on social networking sites - uses & gratifications theory perspective. Journal of Theoretical and Applied Electronic Commerce Research, 15(1), 30-47.
- Kumar, R. G., & Shriram, R. (2019). Sentiment analysis using bi-directional recurrent neural network for Telugu movies. Int J Innov Technol Explor Eng, 9(2), 241-245.
- Kumar, Satheesh, R., et al. (2021). Exploration of sentiment analysis and legitimate artistry for opinion mining. Multimedia Tools and Applications, 1-16.
- Minaee, Shervin, Elham Azimi, & AmirAli Abdolrashidi. (2019). Deep-sentiment: Sentiment analysis using ensemble of CNN and Bi-LSTM models. arXiv preprint arXiv:1904.04206.
- Priya, Balakrishna, G., & Usha Rani, M. (2020). A Framework for Sentiment Analysis of Telugu Tweets. International Journal of Engineering and Advanced Technology (IJEAT), 9(6).
- Rehman, Anwar Ur, et al. (2019). A hybrid CNN-LSTM model for improving accuracy of movie reviews sentiment analysis. Multimedia Tools and Applications, 78(18), 26597-26613.
- Saad, Shihab Elbagir, & Jing Yang. (2019). Twitter sentiment analysis based on ordinal regression. IEEE Access, 7, 163677-163685.
- Shelke, Mahesh B., & Sachin Deshmukh, N. (2020). Recent Advances in Sentiment Analysis of Indian Languages. International Journal of Future Generation Communication and Networking, 13(4), 1656-1675.
- Singh, Vivek Kumar, et al. (2013). Sentiment analysis of movie reviews: A new feature-based heuristic for aspect-level sentiment classification. 2013 International Multi-Conference on Automation, Computing, Communication, Control and Compressed Sensing (IMAC4S). IEEE.
- Suryachandra, Palli, & Venkata Subba Reddy, P. Classification of the Sentiment Value of Natural Language Processing in Telugu Data Using Adabooster Classifier.
- Tran, Huyen Thi Thanh, et al. (2021). Social Media Insights During the COVID-19 Pandemic: Infodemiology Study Using Big Data. JMIR Medical Informatics, 9(7), e27116.
- Urena, Raquel, et al. (2019). A review on trust propagation and opinion dynamics in social networks and group decision making frameworks. Information Sciences, 478, 461-475.
- Yang, Chao, et al. (2019). Aspect-based sentiment analysis with alternating coattention networks. Information Processing & Management, 56(3), 463-478.
- Zhang, Yuebing, et al. (2019). A cost-sensitive three-way combination technique for ensemble learning in sentiment classification. International Journal of Approximate Reasoning, 105, 85-97.