The study on interdependence analysis of product design attributes
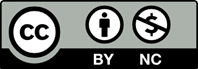
With the improvement of customer awareness, companies have begun developing various diversified designs to meet customer needs. Make the designer face the challenge of multiple customer needs and then increase the difficulty of understanding. Taking a hairdryer as an example, this study applies Fuzzy Interpretive Structural Modeling (FISM) to analyze the interdependence of product design attributes. To effectively clarify the critical design factors and to describe the complex interdependence between each other. The research results show that by analyzing the logical sequence of attributes and transforming them into structured association diagrams and hierarchical diagrams. Structural association diagrams and hierarchical diagrams can help designers identify independent or dependent design factors. It is also possible to identify the interplay between key elements and desired attributes.
- Abuzeinab, A., Arif, M., & Qadri, M.A. (2017). Barriers to MNEs green business models in the UK construction sector: an ISM analysis, Journal of Cleaner Production, 160, 27-37.
- Bellman, R., & Zadeh, L. A. (1970). Decision making in a fuzzy environment, Management Science, 17(4), 141-164.
- Bouzon, M., Govindan, K., & Rodriguez, C.M.T. (2015). Reducing the extraction of minerals: reverse logistics in the machinery manufacturing industry sector in Brazil using ISM approach, Resources Policy, 46, 27-36.
- Chen, S. P., & Wu, W. Y. (2010). A systematic procedure to evaluate an automobile manufacturer distributor partnership, European Journal of Operational Research, 205, 687-698.
- Chen, W. L., & Chiang, Y. M. (2011). A study on innovative product design model using patent information, Journal of Design Science, 14(2), 1-22.
- Govindan, K., Diabat, A., & Madan Shankar, K. (2015). Analyzing the drivers of green manufacturing with fuzzy approach, Journal of Cleaner Production, 96, 182-193.
- Griffin, H., & Hauser, J.R. (1993). The voice of the customer, Marketing Science, 12(1), 1-27.
- Jharkharia, S., & Shankar, R. (2004). IT enablement of supply chains: modeling the enablers, International Journal of Productivity and Performance Management, 53(8), 700-712.
- Jiang, X., Fan, H., Zhang, Y., & Yuan, Z. (2018). Using interpretive structural modeling and fuzzy analytic network process to identify and allocate risks in Arctic shipping strategic alliance, Polar Science, 17, 83-93.
- Jiao, J., & Chen, C. H. (2006). Customer requirement management in product development: a review of research issues, Concurrent Engineering, 14(3), 173-185.
- Karmaker, C. L., Ahmed, T., Ahmed, S., Mithun Ali, S., Moktadir, Md. A., & Kabir, G. (2021). Improving supply chain sustainability in the context of COVID-19 pandemic in an emerging economy: Exploring drivers using an integrated model, Sustainable Production and Consumption, 26, 411-427.
- Khatwani, G., Singh, S.P., Trivedi, A., & Chauhan, A. (2015). Fuzzy-TISM: A fuzzy extension of TISM for group decision making, Global Journal of Flexible Systems Management, 16 (1), 97-112.
- Kota, S., Mishra, R. P, Jasti, N. V. K., & Kale, S. (2021). Sustainable production system critical success factors: an interpretive structural modeling approach, Procedia CIRP, 98, 324-329.
- Kwong, C. K., & Bai, H. (2002). A fuzzy AHP approach to the determination of importance weights of customer requirements in quality function deployment, Journal of Intelligent Manufacturing, 13(5), 367-377.
- Lee, A.H., Kang, H.Y., & Chang, C.C. (2011). An integrated interpretive structural modeling fuzzy analytic network process benefits, opportunities, costs and risks model for selecting technologies, International Journal of Information Technology & Decision Making, 10 (05), 843-871.
- Mandal, A., & Deshmukh, S. G. (1994). Vendor selection using interpretive structural modelling (ISM), International Journal of Operations & Production Management, 14(6), 52-59.
- Myrodia, A., Kristjansdottir, K., & Hvam, L. (2017). Impact of product configuration systems on product profitability and costing accuracy, Computers in Industry, 88, 12-18.
- Narayanan, A.E., Sridharan, R. & Ram Kumar, P.N. (2019). Analyzing the interactions among barriers of sustainable supply chain management practices: A case study, Journal of Manufacturing Technology Management, 30(6), 937-971.
- Panigrahi, S.S., & Sahu, B. (2018). Analysis of interactions among the enablers of green supply chain management using interpretive structural modeling: an Indian perspective, International Journal of Comparative Management, 1 (4), 377-399.
- Raut, R., Gardas, B.B., & Narkhede, B. (2019). Ranking the barriers of sustainable textile and apparel supply chains: an interpretive structural modeling methodology, Benchmarking: An International Journal, 26 (2), 371-394.
- Ravi, V., & Shankar, R. (2005). Analysis of interactions among the barriers of reverse logistics, Technological Forecasting & Social Change, 72, 1011-1029.
- Senecal, S., Kalczynski, P. J., & Nantel, J. (2005). Consumer decision-making process and their online shopping behavior: A click stream analysis, Journal of Business Research, 58(11), 1599-1608.
- Tseng, M. L. (2013). Modeling sustainable production indicators with linguistic preferences, Journal of Cleaner Production, 40, 46-56.
- Valmohammadi, C., & Dashti, S. (2016). Using interpretive structural modeling and fuzzy analytical process to identify and prioritize the interactive barriers of e-commerce implementation, Information & Management, 53 (2), 157-168.
- Violante, M. G., & Vezzetti, E. (2014). A methodology for supporting requirement management tools (RMt) design in the PLM scenario: A user-based strategy, Computers in Industry, 65(7), 1065-1075.
- Warfield, J. N. (1976). Societal Systems: Planning, Policy and Complexity, Wiley Publishers, NY, 1976.
- Warfield, J. N. (1982). Interpretive Structural Modeling (Chapter 5). In S. A. Olsen (Ed.), Group Planning and Problem Solving Methods in Engineering (pp.155-201) (pp.408-411).
- Wu, W.W. (2008). Choosing knowledge management strategies by using a combined ANP and DEMATEL approach, Expert Systems with Applications, 35 (3), 828-835.
- You, N., Kato, Y., & Kitaoka, M. (1994). Numerous date in hierarchy for knowledge concentrated in decision tree, Computers & Industrial Engineering, 27(1-4), 535-538.
- Zadeh, L. A. (1965). Fuzzy sets, Information and Control, 8, 338-353.
- Zayed, E.O. & Yaseen, E.A. (2021). Barriers to sustainable supply chain management implementation in Egyptian industries: an interpretive structural modeling (ISM) approach, Management of Environmental Quality, 32 (6), 1192-1209.