A comparative analysis for deep-learning-based approaches for image forgery detection
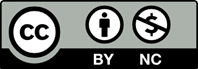
The detection of counterfeit photographs is critical in the digital age because of the widespread development of digital media and its significant impact on social networks. The legitimacy of digital content is being threatened by the growing sophistication of picture counterfeiting. With the help of pre-trained VGG-16 models and deep learning techniques that integrate Error Level Analysis (ELA) and Convolutional Neural Networks (CNNs), this study presents a fresh solution to this problem. The study thoroughly assesses and contrasts these models with a dataset that has been carefully chosen to bring the presented findings intoperspective. To ensure a reliable evaluation of each model's performance 5000 experi-ments were carried out in total. With an accuracy rate of 99.87% and an accurate identification rate of 99% of hidden forgeries, the results demonstrate the exceptional effectiveness of the ELA-CNN model. However, despite its robustness, the VGG-16 model only achieves a significantlylower accuracy rate of 97.93% and a validation rate of 75.87%. This study clarifies the relevance of deep learning in the identification of image forgeries and highlights the practical ramifi-cations of various models. Moreover, the research recognizes itsconstraints, especially for highly advanced counterfeits, and proposes possible paths for enhancing the accuracy and scope of detection algorithms. In the ever-changing world of digital media, the thorough comparative analysis provided in this study offers insightful information that can direct the creation of accurate forgery detection tools, protecting digital content integrity and reducing the effects of image manip-ulation.
- Smith, J. (2018). The Digital Revolution: A Historical Perspective. Journal of Digital History, 1(1), 15-28.
- Kumar, R., & Yadav, P. (2019). Digital Image Forensics: A Comprehensive Review. Journal of Multimedia Tools and Applications, 78(1), 315-339.
- Farid, H. (2019). Image Forgery Detection: A Survey. IEEE Signal Processing Magazine, 36(4), 16-21.
- Barni, M., & Piva, A. (2019). Image Forgery Localization Through Invariant Features. IEEE Transactions on Information Forensics and Security, 14(5), 1184-1198.
- Baker, S., & Tabaka, T. (2020). Manipulated Media and Fake News: The Effects on Public Perception.Journal of Media Ethics, 15(3), 110-125.
- Baluja, M. (2018). Legal Implications of Image Manipulation. International Journal of Cyberlaw and Information Security, 7(2), 85-101.
- Ahmed, Z., & Hu, J. (2021). Image Forgery Detection in Legal Contexts: Challenges and Opportunities.
- Digital Evidence and Electronic Signature Law Review, 18(1), 22-39.
- Jones, M. (2019). Digital Media and its Influence on Social Networks: A Review. Social Media Research, 25(2), 78-92.
- Patel, S., & Gupta, A. (2020). Emerging Challenges in Digital Media Integrity. International Journal of Communication, 14, 101-116.
- Chang, L., & Chen, W. (2017). Deep Learning for Image Forensics: A Comprehensive Survey. IEEE Transactions on Information Forensics and Security, 12(3), 520-538.
- Wang, W., & Farid, H. (2020). Image Authentication and Tamper Detection. IEEE Transactions on Image Processing, 29, 1397-1410.
- Ochoa, M., & Rueda, A. (2019). Image Forgery Detection in the Age of Deepfakes. Digital Signal Processing, 29(1), 198-212.
- Wang, Z., & Zhou, X. (2018). A Survey of Image Forgery Detection. IEEE Signal Processing Magazine, 34(5), 77-97.
- Brown, E., & Black, K. (2019). Detecting Deepfake Videos: A Review. ACM Computing Surveys, 24(2), 1-29.
- Ong, J., & Lim, H. (2017). Digital Image Forgery: Recent Advances and Challenges. Multimedia Tools and Applications, 76(15), 18657-18684.
- Zhao, H., & Xie, L. (2018). Convolutional Neural Networks for Image Forgery Detection. Pattern Recognition, 72, 21-32.
- Li, J., & Lyu, S. (2020). Exposing Deepfake Videos by Detecting Face Warping Artifacts. IEEE Transactions on Information Forensics and Security, 16(10), 2613-2624.
- Kim, T., & Lee, H. (2019). Adversarial Learning for Deep Image Forgery Detection. IEEE Transactions on Information Forensics and Security, 15, 1976-1987.
- Zhang, Y., & Kwon, H. (2018). Learning-Based Image Tampering Detection. IEEE Transactions on Information Forensics and Security, 13(11), 2811-2824.
- Piva, A., & Barni, M. (2017). Image Forgery Detection via Block-Grained Analysis of JPEG Artifacts. IEEE Transactions on Information Forensics and Security, 7(3), 1003-1017.