Enhancing digital security using Signa-Deep for online signature verification and identity authentication
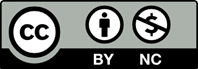
In the contemporary digital realm, the utilization of online services has surged, facilitated by the seamless integration of deep learning technology, which is paramount in applications demanding precision and efficiency. A pivotal use case in this context is online handwritten signature verification, where the need for exceptional accuracy is indisputa-ble. This paper introduces 'Signa-Deep,' an innovative approach designed to address the challenge of online signature verification and the determination of an individual's authorization status. The study explores a range of methodologies, including Convolutional Neural Networks (CNN), Long Short-Term Memory (LSTM), GoogleNet, and MobileNet, to discern the authenticity of signatures and affirm the identity of the signatory. The results of our proposed method are promising, showcasing its potential to significantly enhance the security of digital transactions and identity veri-fication processes. In summary, 'Signa-Deep' harnesses deep learning technology to bolster the accuracy and reliability of online signature verification, thereby contributing to the overall robustness of digital interactions and identity val-idation processes.
- Larijani, A., & Dehghani, F. (2024). Computationally Efficient Method for Increasing Confidentiality in Smart Electricity Networks. *Electronics*, 13(1), 170. https://doi.org/10.3390/electronics13010170.
- Shekar, B. H. & Bharathi, R. K. (2011). Eigen-signature: A Robust and an Efficient Offline Signature Verification Algorithm. In *2011 International Conference on Recent Trends in Information Technology (ICRTIT)*, Chennai, India, 134-138. doi: 10.1109/ICRTIT.2011.5972461.
- Shekar, B. H., Abraham, W. & Pilar, B. (2022). Offline Signature Verification Using CNN and SVM Classifier. In *2022 IEEE 7th International Conference on Recent Advances and Innovations in Engineering (ICRAIE)*, Mangalore, India, 304-307. doi: 10.1109/ICRAIE56454.2022.10054336.
- Low, C. Y., Teoh, A. B. J. & Tee, C. (2007). A Preliminary Study on Biometric Watermarking for Offline Handwritten Signature. In *2007 IEEE International Conference on Telecommunications and Malaysia International Conference on Communications*, Penang, Malaysia, 691-696. doi: 10.1109/ICTMICC.2007.4448568.
- Chang, S. J., & Wu, T. R. (2023). Development of a Signature Verification Model Based on a Small Number of Samples. *Signal, Image and Video Processing*, 2023, 1-10.
- Falahati, D., Helfrush, M., Danyali, H. & Rashidpour, M. (2011). Static Signature Verification for Farsi and Arabic Signatures Using Dynamic Time Warping. In *2011 19th Iranian Conference on Electrical Engineering*, Tehran, Iran, 1-1.
- Vajpai, J., Arun, J.B., & Vajpai, I. (2013). Dynamic Signature Verification for Secure Retrieval of Classified Information. In *2013 Fourth National Conference on Computer Vision, Pattern Recognition, Image Processing and Graphics (NCVPRIPG)*, Jodhpur, India, 1-4. doi: 10.1109/NCVPRIPG.2013.6776170.
- Anatska, K., & Shekaramiz, M. (2022). Offline Signature Verification: A Study on Total Variation versus CNN. In *2022 Intermountain Engineering, Technology and Computing (IETC)*, Orem, UT, USA, 1-6. doi: 10.1109/IETC54973.2022.9796924.
- Larijani, A., & Dehghani, F. (2024). An Efficient Optimization Approach for Designing Machine Models Based on Combined Algorithm. *FinTech*, 3(1), 40-54. https://doi.org/10.3390/fintech3010003.
- Fayyaz, M., Saffar, M. H., SabokrouM., Hoseini, M. &Fathy, M. (2015).Online Signature Verification Based on Feature Representation. 2015 The International Symposium on Artificial Intelligence and Signal Processing (AISP), Mashhad, Iran, 2015, pp. 211-216. doi: 10.1109/AISP.2015.7123528.
- Yapici, M., Tekerek, M. A., & Topaloglu, N. (2018). Convolutional Neural Network Based Offline Signature Verification Application. In *2018 International Congress on Big Data, Deep Learning and Fighting Cyber Terrorism (IBIGDELFT)*, Ankara, Turkey, 30-34. doi: 10.1109/IBIGDELFT.2018.8625290.
- Abbas, N., & Chibani, Y. (2012). SVM-DSmT Combination for Off-Line Signature Verification. In *2012 International Conference on Computer, Information and Telecommunication Systems (CITS)*, Amman, Jordan, 1-5. doi: 10.1109/CITS.2012.6220365.
- Sharma, N., Gupta, S., Mehta, P., Cheng, X., Shankar, A., Singh, P., & Nayak, S. R. (2022). Offline Signature Verification Using a Deep Neural Network with Application to Computer Vision. *Journal of Electronic Imaging*, 31(4), 041210. doi: 10.1117/1.JEI.31.4.041210.
- Shapran, O., & Fairhurst, M. C. (2009). Enhancing Signature Verification Using Alternative Handwriting Semantics. In *3rd International Conference on Imaging for Crime Detection and Prevention (ICDP 2009)*, London, 1-6. doi: 10.1049/ic.2009.0242.
- Sonawane, R. C., & Patil, M. E. (2012). An Effective Stroke Feature Selection Method for Online Signature Verification. In *2012 Third International Conference on Computing, Communication and Networking Technologies (ICCCNT'12)*, Coimbatore, India, 1-6. doi: 10.1109/ICCCNT.2012.6395926.
- Suganthe, R. C., Geetha, M., Sreekanth, G. R., Manjunath, R., Krishna, S. M., & Balaji, P. M. (2022). Performance Evaluation of Convolutional Neural Network Based Models on Signature Verification System. In *2022 International Conference on Computer Communication and Informatics (ICCCI)*, Coimbatore, India, 1-6. doi: 10.1109/ICCCI54379.2022.9741030.
- Choupanzadeh, R., & Zadehgol, A. (2023). A Deep Neural Network Modeling Methodology for Efficient EMC Assessment of Shielding Enclosures Using MECA-Generated RCS Training Data. *IEEE Transactions on Electromagnetic Compatibility*, 65(6), 1782-1792. doi: 10.1109/TEMC.2023.3316916.
- Wijewickrama, R., Abbasihafshejani, M., Maiti, A., & Jadliwala, M. (2023). OverHear: Headphone-Based Multi-Sensor Keystroke Inference. *arXiv preprint arXiv:2311.02288*, 2023.
- Ren, Y., Wang, C., Chen, Y., Chuah, M. C., & Yang, J. (2020). Signature Verification Using Critical Segments for Securing Mobile Transactions. *IEEE Transactions on Mobile Computing*, 19(3), 724-739. doi: 10.1109/TMC.2019.2897657.