A systematic meta-analysis on the role of artificial intelligence and machine learning in detection of gynaecological disorders
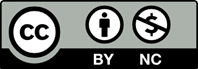
Globally, one of the major concerns in women’s health issues is gynecological disorders such as cancer, which needs to be observed at its early stage. With traditional approaches, it is quite difficult to detect such disorders at its early stages. Therefore, more advanced tools need to be integrated. This paper focuses the advancements of artificial intelligence (AI) and machine learning (ML), exploring their potential in the early detection and diagnosis of these disorders. This paper presents a systematic meta-analysis of AI/ML approaches employed in the diagnosis of gynecological disorders using medical imaging modalities such as magnetic resonance imaging (MRI), ultrasound, etc. The flow for systematic meta-analysis is based on designing the research objective, selection andsearching approach with inclusion and exclusion strategy; quality assessment is performed then; and finally, discussion of interpretations is also presented. This paper investigates how ML algorithms can extract characteristics from MRI images and how to use ML to extract and recognize the features from medical images such as MRI, ultrasound, computed tomography (CT) scans, etc. for early detection of gynecological tumors and provision of more personalized risk assessment. However, it is observed that there is a significant impact of advancement of AI/ML on medical technology in the future. Therefore, this paper presents a significant contribution for future medical applications and innovations.
- Adweb, K. M. A., Cavus, N., & Sekeroglu, B. (2021). Cervical Cancer Diagnosis Using Very Deep Networks Over Different Activation Functions. IEEE Access, 9, 46612–46625.https://doi.org/10.1109/ACCESS.2021.3067195
- Akazawa, M., & Hashimoto, K. (2021). Artificial intelligence in gynecologic cancers: Current status and future challenges -A systematic review. Artificial Intelligence in Medicine, 120, 102164. https://doi.org/10.1016/j.artmed.2021.102164
- Arezzo, F., Cormio, G., La Forgia, D., Santarsiero, C. M., Mongelli, M., Lombardi, C., Cazzato, G., Ci-cinelli, E., & Loizzi, V. (2022). A machine learning approach applied to gynecological ultrasound to predict progression-free survival in ovarian cancer patients. Archives of Gynecology and Obstetrics, 306(6), 2143–2154. https://doi.org/10.1007/s00404-022-06578-1
- Arora, A., Tripathi, A., & Bhan, A. (2021). Classification of Cervical Cancer Detection using Ma-chine Learning Algorithms. 2021 6th International Conference on Inventive Computation Technologies (ICICT), 827–835. https://doi.org/10.1109/ICICT50816.2021.9358570
- Basij, M., Yan, Y., Alshahrani, S., Winer, I., Bur-meister, J., Dominello, M., & Mehrmohammadi, M. (2018). Development of an Ultrasound and Photoacoustic Endoscopy System for Imaging of Gynecological Disorders. 2018 IEEE International Ultrasonics Symposium(IUS), 1–4. https://doi.org/10.1109/ULTSYM.2018.8579788
- Baydoun, A., Xu, K. E., Heo, J. U., Yang, H., Zhou, F., Bethell, L. A., Fredman, E. T., Ellis, R. J., Podder, T. K., Traughber, M. S., Paspulati, R. M., Qian, P., Traughber, B. J., & Muzic, R. F. (2021). Synthetic CT Generation of the Pelvis in Patients with Cervical Cancer: A Single Input Approach Using Generative Adversarial Network. IEEE Access: Practical Innovations, Open Solutions, 9, 17208–17221. https://doi.org/10.1109/access.2021.3049781
- Behboodi, B., Rivaz, H., Lalondrelle, S., & Harris, E. (2021). Automatic 3D Ultrasound Segmentation of Uterus Using Deep Learning. IEEE International Ultrasonics Symposium, IUS, 3–6. https://doi.org/10.1109/IUS52206.2021.9593671
- Bharati, S., Podder, P., & Hossain Mondal, M. R. (2020). Diagnosis of Polycystic Ovary Syndrome Using Machine Learning Algorithms. 2020 IEEE Region 10 Symposium (TENSYMP), 1486–1489. https://doi.org/10.1109/TENSYMP50017.2020.9230932
- Bharati, S., Podder, P., Mondal, M. R., Prasath, S., & Gandhi, N. (2022). Ensemble Learning for Da-ta-Driven Diagnosis of Polycystic Ovary Syn-drome. 1250–1259. https://doi.org/10.1007/978-3-030-96308-8_116
- Bhattacharjee, S., Singh, Y. J., & Ray, D. (2017). Comparative performance analysis of machine learning classifiers on ovarian cancer dataset. 2017 Third International Conference on Research in Computational Intelligence and Communication Networks (ICRCICN), 213–218. https://doi.org/10.1109/ICRCICN.2017.8234509
- Bhavani, C. H., & Govardhan, A. (2023). Cervical cancer prediction using stacked ensemble algo-rithm with SMOTE and RFERF. Materials Today: Proceedings, 80, 3451–3457.https://doi.org/https://doi.org/10.1016/j.matpr.2021.07.269
- Bnouni, N., Amor, H. Ben, Rekik, I., Rhim, M. S., Solaiman, B., & Amara, N. E. Ben. (2021). Boosting CNN Learning by Ensemble Image Pre-processing Methods for Cervical Cancer Seg-mentation. 2021 18th International Mul-ti-Conference on Systems, Signals & Devices (SSD), 264–269. https://doi.org/10.1109/SSD52085.2021.9429422
- Chang, C.-C., Chen, H.-H., Chang, Y.-C., Yang, M.-Y., Lo, C.-M., Ko, W.-C., Lee, Y.-F., Liu, K.-L., & Chang, R.-F. (2017). Computer-aided diagnosis of liver tumors on computed tomography images. Computer Methods and Programs in Biomedicine, 145, 45–51. https://doi.org/https://doi.org/10.1016/j.cmpb.2017.04.008
- Chauhan, N. K., & Singh, K. (2021). Impact of Vari-ation in Number of Channels in CNN Classifica-tion model for Cervical Cancer Detection. 2021 9th International Conference on Reliability, Infocom Technologies and Optimization (Trends and Future Directions) (ICRITO), 1–6. https://doi.org/10.1109/ICRITO51393.2021.9596366
- Chen, C., Wang, Y., Niu, J., Liu, X., Li, Q., & Gong, X. (2021). Domain Knowledge Powered Deep Learning for Breast Cancer Diagnosis Based on Contrast-Enhanced Ultrasound Videos. IEEE Transactions on Medical Imaging, 40(9), 2439–2451. https://doi.org/10.1109/TMI.2021.3078370
- Chitra, P., Srilatha, K., Sumathi, M., Jayasudha, F. V, Bernatin, T., & Jagadeesh, M. (2023). Classification of Ultrasound PCOS Image using Deep Learning based Hybrid Models. 2023 Second International Conference on Electronics and Renewable Systems (ICEARS), 1389–1394. https://doi.org/10.1109/ICEARS56392.2023.10085400
- Coffin, T., Wray, J., Sah, R., Maj, M., Nath, R., Nauhria, S., Maity, S., & Nauhria, S. (2023). A Review and Meta-Analysis of the Prevalence and Health Impact of Polycystic Ovary Syndrome Among Medical and Dental Students. Cureus, 15(6), e40141. https://doi.org/10.7759/cureus.40141
- Constantinou, I. P., Koumourou, C. A., Neofytou, M. S., Tanos, V., Pattichis, C. S., & Kyriakou, E. C. (2009). An integrated CAD system facilitating the endometrial cancer diagnosis. 2009 9th Interna-tional Conference on Information Technology and Applications in Biomedicine, 1–5. https://doi.org/10.1109/ITAB.2009.5394424
- Coşar Soğukkuyu, D. Y., & Ata, O. (2022). Diagnosing Cervical Cancer Using Machine Learning Methods. 2022 International Congress on Hu-man-Computer Interaction, Optimization and Robotic Applications (HORA), 1–3. https://doi.org/10.1109/HORA55278.2022.9800033
- de Carvalho Filho, A. O., Silva, A. C., Cardoso de Paiva, A., Nunes, R. A., & Gattass, M. (2017). Computer-Aided Diagnosis of Lung Nodules in Computed Tomography by Using Phylogenetic Diversity, Genetic Algorithm, and SVM. Journal of Digital Imaging, 30(6), 812–822. https://doi.org/10.1007/s10278-017-9973-6
- Denny, A., Raj, A., Ashok, A., Ram, C. M., & George, R. (2019). i-HOPE: Detection and Prediction System For Polycystic Ovary Syndrome (PCOS) Using Machine Learning Techniques. TENCON 2019 -2019 IEEE Region 10 Conference (TENCON), 673–678. https://doi.org/10.1109/TENCON.2019.8929674
- Gao, Y., Zeng, S., Xu, X., Li, H., Yao, S., Song, K., Li, X., Chen, L., Tang, J., Xing, H., & Yu, Z. (2022). Articles Deep learning-enabled pelvic ultrasound images for accurate diagnosis of ovarian cancer in China: a retrospective, multicentre, diagnosticstudy. 179–187. https://doi.org/10.1016/S2589-7500(21)00278-8
- Ghoneim, A., Muhammad, G., & Hossain, M. S. (2020). Cervical cancer classification using convolutional neural networks and extreme learning machines. Future Generation Computer Systems, 102, 643–649. https://doi.org/https://doi.org/10.1016/j.future.2019.09.015
- Goudarzi, S., Whyte, J., Boily, M., Towers, A., Kilgour, R. D., & Rivaz, H. (2023). Segmentation of Arm Ultrasound Images in Breast Cancer-Related Lymphedema: A Database and Deep Learning Algorithm. IEEE Transactions on Bio-Medical Engineering, 70(9), 2552–2563. https://doi.org/10.1109/TBME.2023.3253646
- Guo, Z., Li, X., Huang, H., Guo, N., & Li, Q. (2019). Deep Learning-Based Image Segmentation on Multimodal Medical Imaging. IEEE Transactions on Radiation and Plasma Medical Sciences, 3(2), 162–169. https://doi.org/10.1109/TRPMS.2018.2890359
- Harish, K. P., Dhivyanchali, M. N., Devi, K. N., Krishnamoorthy, N., Dhana Sree, R., & Dharanidharan, R. (2023). Smart Diagnostic System For Early Detection And Prediction Of Polycystic Ovary Syndrome. 2023 International Conference on Computer Communication and Informatics (ICCCI), 1–6. https://doi.org/10.1109/ICCCI56745.2023.10128560
- Hou, X., Shen, G., Zhou, L., Li, Y., Wang, T., & Ma, X. (2022). Artificial Intelligence in Cervical Cancer Screening and Diagnosis. Frontiers in Oncology, 12, 851367. https://doi.org/10.3389/fonc.2022.851367
- Hu, D., Jian, J., Li, Y., & Gao, X. (2023). Deep learning-based segmentation of epithelial ovarian cancer on T2-weighted magnetic resonance im-ages. Quantitative Imaging in Medicine and Sur-gery, 13(3), 1464–1477. https://doi.org/10.21037/qims-22-494
- Hyun, D., Abou-Elkacem, L., Bam, R., Brickson, L. L., Herickhoff, C. D., & Dahl, J. J. (2020). Nondestructive Detection of Targeted Microbubbles Using Dual-Mode Data and Deep Learning for Real-Time Ultrasound Molecular Imaging. IEEE Transactions on MedicalImaging, 39(10), 3079–3088. https://doi.org/10.1109/TMI.2020.2986762
- Jiang, X., Li, J., Kan, Y., Yu, T., Chang, S., Sha, X., Zheng, H., Luo, Y., & Wang, S. (2021). MRI Based Radiomics Approach With Deep Learning for Prediction of Vessel Invasion in Early-Stage Cervical Cancer. IEEE/ACM Transactions on Computational Biology and Bioinformatics, 18(3), 995–1002. https://doi.org/10.1109/TCBB.2019.2963867
- Kajala, A., & Jain, V. K. (2020). Diagnosis of Breast Cancer using Machine Learning Algorithms-A Review. 2020 International Conference on Emerging Trends in Communication, Control and Computing (ICONC3), 1–5. https://doi.org/10.1109/ICONC345789.2020.9117320
- Kapruwan, A., Sharma, S., & Goyal, H. R. (2023). Artificial Intelligence Enabled Diagnostic Digital Cytopathology System for Cervical Intraepithelial Neoplasia Detection: Advantages and Challenges. 2023 Third International Conference on Artificial Intelligence and Smart Energy (ICAIS), 669–674. https://doi.org/10.1109/ICAIS56108.2023.10073850
- Kasture, K. R., Sayankar, B. B., & Matte, P. N. (2021). Multi-class Classification of Ovarian Cancer from Histopathological Images using Deep Learning -VGG-16. 2021 2nd Global Conference for Advancement in Technology (GCAT), 1–6. https://doi.org/10.1109/GCAT52182.2021.9587760
- Keymasi, M., Mishra, V., Aslan, S., & Asem, M. (2018). Theoretical Assessment of Cervical Cancer Using Machine Learning Methods Based on Pap-Smear Test. 1367–1373. https://doi.org/10.1109/IEMCON.2018.8615041
- Khanna, V. V., Chadaga, K., Sampathila, N., Prabhu, S., Bhandage, V., & Hegde, G. K. (2023). A Distinctive Explainable Machine Learning Frame-work for Detection of Polycystic Ovary Syn-drome. Applied System Innovation, 6(2). https://doi.org/10.3390/asi6020032
- Khuriwal, N., & Mishra, N. (2018). Breast Cancer Diagnosis Using Deep Learning Algorithm. 2018 International Conference on Advances in Computing, Communication Control and Networking (ICACCCN), 98–103. https://doi.org/10.1109/ICACCCN.2018.8748777
- Kiruthika, V., Sathiya, S., & Ramya, M.M. (2020). Machine learning based ovarian detection in ultrasound images. International Journal of Advanced Mechatronic Systems, 8, 75. https://doi.org/10.1504/IJAMECHS.2020.111306
- Kurnianingsih, Allehaibi, K. H. S., Nugroho, L. E., Widyawan, Lazuardi, L., Prabuwono, A. S., & Mantoro, T. (2019). Segmentation and Classification of Cervical Cells Using Deep Learning. IEEE Access, 7, 116925–116941. https://doi.org/10.1109/ACCESS.2019.2936017
- Li, Y., Chen, J., Xue, P., Tang, C., Chang, J., Chu, C., Ma, K., Li, Q., Zheng, Y., & Qiao, Y. (2020). Computer-Aided Cervical Cancer Diagnosis Using Time-Lapsed Colposcopic Images. IEEE Transactions on Medical Imaging, 39(11), 3403–3415. https://doi.org/10.1109/TMI.2020.2994778
- Lingappa, E., & Parvathy, L. R. (2023). Image Classification with Deep Learning Methods for Detecting and Staging Bone Cancer from MRI. 2023 International Conference on Networking and Communications (ICNWC), 1–8. https://doi.org/10.1109/ICNWC57852.2023.10127368
- Liu, X., Xiao, Z., Song, Y., Zhang, R., Li, X., & Du, Z. (2021). A Machine Learning-Aided Framework to Predict Outcomes of Anti-PD-1 Therapy for Patients With Gynecological Cancer on Incomplete Post-Marketing Surveillance Dataset. IEEE Access, 9, 120464–120480. https://doi.org/10.1109/ACCESS.2021.3107498
- Marques, S., Carvalho, C., Peixoto, C., Pignatelli, D., Beires, J., Silva, J., & Campilho, A. (2019). Seg-mentation of gynaecological ultrasound images using different U-Net based approaches. 2019 IEEE International Ultrasonics Symposium (IUS), 1485–1488. https://doi.org/10.1109/ULTSYM.2019.8925948
- Mehrotra, P., Chatterjee, J., Chakraborty, C., Ghoshdastidar, B., & Ghoshdastidar, S. (2011). Automated screening of Polycystic Ovary Syn-drome using machine learning techniques. 2011 Annual IEEE India Conference, 1–5. https://doi.org/10.1109/INDCON.2011.6139331
- Nasim, S., Almutairi, M. S., Munir, K., Raza, A., & Younas, F. (2022). A Novel Approach for Poly-cystic Ovary Syndrome Prediction Using Ma-chine Learning in Bioinformatics. IEEE Access, 10, 97610–97624. https://doi.org/10.1109/ACCESS.2022.3205587
- Nishio, M., & Nagashima, C. (2017). Computer-aided Diagnosis for Lung Cancer: Usefulness of Nodule Heterogeneity. Academic Radiology, 24(3), 328–336. https://doi.org/https://doi.org/10.1016/j.acra.2016.11.007
- Nithya, B., & Ilango, V. (2020). Machine Learning Aided Fused Feature Selection based Classification Framework for Diagnosing Cervical Cancer. 2020 Fourth International Conference on Computing Methodologies and Communication (ICCMC), 61–66. https://doi.org/10.1109/ICCMC48092.2020.ICCMC-00011
- Poorani, B. & Khilar, R. (2023). Classification of PCOS Using Machine Learning Algorithms Based on Ultrasound Images of Ovaries. 2023 Eighth International Conference on Science Technology Engineering and Mathematics (ICONSTEM), 1–7. https://api.semanticscholar.org/CorpusID:259101230
- Prapty, A. S., & Shitu, T. T. (2020). An Efficient Decision Tree Establishment and Performance Analysis with Different Machine Learning Approaches on Polycystic Ovary Syndrome. 2020 23rd International Conference on Computer and Information Technology (ICCIT), 1–5. https://doi.org/10.1109/ICCIT51783.2020.9392666
- Prasher, S., & Nelson, L. (2023). Follicle Prediction for Polycystic Ovary Syndrome Diagnosis from Ovarian Ultrasound Images using CNN. 2023 10th International Conference on Computing for Sustainable Global Development (INDIACom), 789–793.
- Rahman, M. A., Muniyandi, R. C., Islam, K. T., & Rahman, M. M. (2019). Ovarian Cancer Classification Accuracy Analysis Using 15-Neuron Artificial Neural Networks Model. 2019 IEEE Student Conference on Research and Development (SCOReD), 33–38. https://doi.org/10.1109/SCORED.2019.8896332
- Ratul, I. J., Al-Monsur, A., Tabassum, B., Ar-Rafi, A. M., Nishat, M. M., & Faisal, F. (2022). Early risk prediction of cervical cancer: A machine learning approach. 2022 19th International Conference on Electrical Engineering/Electronics, Computer, Telecommunications and Information Technology (ECTI-CON), 1–4. https://doi.org/10.1109/ECTI-CON54298.2022.9795429
- Ray, S. (2019). A Quick Review of Machine Learning Algorithms. 2019 International Conference on Machine Learning, Big Data, Cloud and Parallel Computing (COMITCon), 35–39. https://doi.org/10.1109/COMITCon.2019.8862451
- Ruchitha, P. J., Sai Richitha, Y., Kodipalli, A., & Martis, R. J. (2021). Segmentation of Ovarian Cancer using Active Contour and Random Walker Algorithm. 2021 5th International Conference on Electrical, Electronics, Communication, Computer Technologies and Optimization Techniques (ICEECCOT), 238–241. https://doi.org/10.1109/ICEECCOT52851.2021.9707939
- Senthilkumar, G., Ramakrishnan, J., Frnda, J., Manikandan, R., Gupta, D., Tiwari, P., Mohammad, S., & Mohammed, M. (2021). Incorporating Artificial Fish Swarm in Ensemble Classification Framework for Recurrence Prediction of Cervical Cancer. IEEE Access, PP, 1. https://doi.org/10.1109/ACCESS.2021.3087022
- Shrestha, P., Poudyal, B., Yadollahi, S., E Wright, D., V Gregory, A., D Warner, J., Korfiatis, P., C Green, I., L Rassier, S., Mariani, A., Kim, B., Laugh-lin-Tommaso, S. K., & L Kline, T. (2022). A systematic review on the use of artificial intelligence in gynecologic imaging -Background, state of the art, and future directions. Gynecologic Oncology, 166(3), 596–605. https://doi.org/10.1016/j.ygyno.2022.07.024
- Srivastava, S., Kumar, P., Chaudhry, V., & Singh, A. (2020). Detection of Ovarian Cyst in Ultrasound Images Using Fine-Tuned VGG-16 Deep Learning Network. SN Computer Science, 1(2), 81. https://doi.org/10.1007/s42979-020-0109-6
- Subramanian, M., Cho, J., Sathishkumar, V. E., & Naren, O. S. (2023). Multiple Types of Cancer Classification Using CT/MRI Images Based on Learning Without Forgetting Powered Deep Learning Models. IEEE Access, 11, 10336–10354. https://doi.org/10.1109/ACCESS.2023.3240443
- Suha, S. A., & Islam, M. N. (2022). An extended machine learning technique for polycystic ovary syndrome detection using ovary ultrasound im-age. Scientific Reports, 12(1), 17123. https://doi.org/10.1038/s41598-022-21724-0
- Swapnarekha, H., Dash, P. B., Nayak, J., & Routray, A. R. (2023). An Optimistic Bayesian Optimization Based Extreme Learning Machine for Poly-cystic Ovary Syndrome Diagnosis. In J. Nayak, A. K. Das, B. Naik, S. K. Meher, & S. Brahnam (Eds.), Nature-Inspired Optimization Methodologies in Biomedical and Healthcare (pp. 175–193). Springer International Publishing. https://doi.org/10.1007/978-3-031-17544-2_8
- Taleb, N., Mehmood, S., Zubair, M., Naseer, I., Mago, B., & Nasir, M. U. (2022). Ovary Cancer Diag-nosing Empowered with Machine Learning. 2022 International Conference on Business Analytics for Technology and Security (ICBATS), 1–6. https://doi.org/10.1109/ICBATS54253.2022.9759010
- Tanaka, Y. O., Okada, S., Satoh, T., Matsumoto, K., Oki, A., Saida, T., Yoshikawa, H., & Minami, M. (2016). Differentiation of epithelial ovarian cancer subtypes by use of imaging and clinical data: a detailed analysis. Cancer Imaging: The Official Publication of the International Cancer Imaging Society, 16, 3. https://doi.org/10.1186/s40644-016-0061-9
- Tiwari, S., Kane, L., Koundal, D., Jain, A., Alhudhaif, A., Polat, K., Zaguia, A., Alenezi, F., & Althubiti, S. A. (2022). SPOSDS: A smart Polycystic Ovary Syndrome diagnostic system using machine learning. Expert Systems with Applications, 203, 117592. https://doi.org/https://doi.org/10.1016/j.eswa.2022.117592
- Tripathi, A., Arora, A., & Bhan, A. (2021). Classification of cervical cancer using Deep Learning Algorithm. 2021 5th International Conference on Intelligent Computing and Control Systems (ICICCS), 1210–1218. https://doi.org/10.1109/ICICCS51141.2021.9432382
- Visalaxi, S., Punnoose, D., & Muthu, T. S. (2021). An Analogy of Endometriosis Recognition Using Machine Learning Techniques. 2021 Third International Conference on Intelligent Communication Technologies and Virtual Mobile Net-works (ICICV), 739–746. https://doi.org/10.1109/ICICV50876.2021.9388403
- Wang, G., Zhan, H., Luo, T., Kang, B., Li, X., Xi, G., Liu, Z., & Zhuo, S. (2023). Automated Ovarian Cancer Identification Using End-to-End Deep Learning and Second Harmonic Generation Im-aging. IEEE Journal of Selected Topics in Quan-tum Electronics, 29(4: Biophotonics), 1–9. https://doi.org/10.1109/JSTQE.2022.3228567
- Wang, R., Cai, Y., Lee, I. K., Hu, R., Purkayastha, S., Pan, I., Yi, T., Tran, T. M. L., Lu, S., Liu, T., Chang, K., Huang, R. Y., Zhang, P. J., Zhang, Z., Xiao, E., Wu, J., & Bai, H. X. (2021). Evaluation of a convolutional neural network for ovarian tumor differentiation based on magnetic resonance im-aging. European Radiology, 31(7), 4960–4971. https://doi.org/10.1007/s00330-020-07266-x
- Wang, R., Gu, Z., Wang, Y., Yin, X., Liu, W., Chen, W., Huang, Y., Wu, J., Yang, S., Feng, L., Zhou, L., Li, L., Di, W., Pu, X., Huang, L., & Qian, K. (2022). A “One-Stop Shop” Decision Tree for Diagnosing and Phenotyping Polycystic Ovarian Syndrome on Serum Metabolic Fingerprints. Advanced Functional Materials, 32(45), 2206670. https://doi.org/10.1002/ADFM.202206670
- Zahras, D., & Rustam, Z. (2018). Cervical Cancer Risk Classification Based on Deep Convolutional Neural Network. 2018 International Conference on Applied Information Technology and Innova-tion (ICAITI), 149–153. https://doi.org/10.1109/ICAITI.2018.8686767
- Zhang, L., Huang, J., & Liu, L. (2023). Retraction Note: Improved Deep Learning Network Based in Combination with Cost-sensitive Learning for Early Detection of Ovarian Cancer in Color Ul-trasound Detecting System. Journal of Medical Systems, 47(1), 20. https://doi.org/10.1007/s10916-023-01915-6
- Zhang, Z., & Han, Y. (2020). Detection of Ovarian Tumors in Obstetric Ultrasound Imaging Using Logistic Regression Classifier with an Advanced Machine Learning Approach. IEEE Access, 8, 44999–45008. https://doi.org/10.1109/ACCESS.2020.2977962
- Zhou, H., Wang, S., Zhang, T., Liu, D., & Yang, K. (2021). Ultrasound image analysis technology under deep belief networks in evaluation on the effects of diagnosis and chemotherapy of cervical cancer. The Journal of Supercomputing, 77(4), 4151–4171. https://doi.org/10.1007/s11227-020-03421-9
- Zhou, J., Zeng, Z. Y., & Li, L. (2020). Progress of Artificial Intelligence in Gynecological Malignant Tumors. Cancer Management and Research, 12, 12823–12840. https://doi.org/10.2147/CMAR.S279990.