Prostate cancer prediction using machine learning techniques
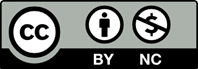
Prostate cancer (PCa) is currently the most frequently diagnosed cancer in men in industrialized nations and ranks as the second leading cause of male cancer-related deaths globally, early detection is crucial. Originating in the walnut-shaped gland beneath the bladder, PCa poses a significant risk when not identified in its early stages. The diagnostic process, requiring expertise from radiologists, pathologists, and physicians, is time-consuming and introduces varia-bility, potentially leading to delayed or incorrect diagnoses. This underscores the need for efficient and reliable diag-nostic tools in addressing the escalating challenge of PCa diagnosis.This study addresses the critical challenge of PCa diagnosis by employing a comprehensive approach involving feature selection methods and model performance eval-uation. Utilizing a PCa dataset from Kaggle, consisting of 100 patient observations with eight independent features and a binary diagnosis result, the study explores the nuanced nature of feature relevance in PCa classification. Com-parative analyses of Principal Component Analysis (PCA) and ReliefF feature selection methods reveal the limitations of PCA's emphasis on a dominant feature, while ReliefF, incorporating a distributed set of features, demonstrates improved modelaccuracy and stability. The Random Forest (RF) model, selected through meticulous parameter tuning, achieves an impressive 95% accuracy by leveraging a substantial number of estimators, limited tree depth, and bal-anced sample splitting. The findings underscore the crucial interplay between feature selection methods and model parameters in optimizing the accuracy and reliability of PCa classification models. Given the anticipated rise in PCa incidence, this research contributes valuable insights for enhancing diagnostic efficiency and addressing the challenges posed by traditional diagnostic procedures.
- ACS (American Cancer Society). (2023). *Survival Rates for Prostate Cancer*. Atlanta, GA, USA.
- Alhanaya, M., & Ateyeh Al-Shqeerat, K. H. (2023). Performance Analysis of Intrusion Detection System in the IoT Environment Using Feature Selection Technique. *Intelligent Automation & Soft Computing*, 36(3).
- Araste, Z., Sadighi, A., & Jamimoghaddam, M. (2023). Fault diagnosis of a centrifugal pump using electrical signature analysis and support vector machine. *Journal of Vibration Engineering & Technologies*, 11(5), 2057-2067.
- Araujo, W. B., Santana, E. E., Sousa, N. P., Junior, C. M., Allan Filho, K. D. B., Moura, G. L., ... & Silva, F. C. (2023). Method to aid the diagnosis of prostate cancer using machine learning and clinical data.
- Chen, S., Jian, T., Chi, C., Liang, Y., Liang, X., Yu, Y., ... & Lu, J. (2022). Machine learning-based models enhance the prediction of prostate cancer. *Frontiers in Oncology*, 12, 941349.
- De Vos, I. I., Luiting, H. B., & Roobol, M. J. (2023). Active Surveillance for Prostate Cancer: Past, Current, and Future Trends. *Journal of Personalized Medicine*, 13(4), 629.
- Deka, M. J., Kalita, P., Das, D., Kamble, A. D., Bora, B. J., Sharma, P., & Medhi, B. J. (2023). An approach towards building robust neural networks models using multilayer perceptron through experimentation on different photovoltaic thermal systems. *Energy Conversion and Management*, 292, 117395.
- Erdem, E., & Bozkurt, F. (2021). A comparison of various supervised machine learning techniques for prostate cancer prediction. *Avrupa Bilim ve Teknoloji Dergisi*, (21), 610-620.
- Gavade, A. B., Nerli, R., Kanwal, N., Gavade, P. A., Pol, S. S., & Rizvi, S. T. H. (2023). Automated diagnosis of prostate cancer using mpmri images: A deep learning approach for clinical decision support. *Computers*, 12(8), 152.
- Laabidi, A., & Aissaoui, M. (2020, April). Performance analysis of Machine learning classifiers for predicting diabetes and prostate cancer. In *2020 1st International Conference on Innovative Research in Applied Science, Engineering and Technology (IRA-SET)* (pp. 1-6). IEEE.
- Molla, M. I., Jui, J. J., Rana, H. K., & Podder, N. K. (2023, January). Machine Learning Algorithms for the Prediction of Prostate Cancer. In *Proceedings of International Conference on Information and Communication Technology for Development: ICICTD 2022* (pp. 471-482). Singapore: Springer Nature Singapore.
- Naeem, A., Khan, A. H., u din Ayubi, S., & Malik, H. (2023). Predicting the Metastasis Ability of Prostate Cancer using Machine Learning Classifiers. *Journal of Computing & Biomedical Informatics*, 4(02), 1-7.
- Podgorelec, V., Kokol, P., Stiglic, B., & Rozman, I. (2002). Decision trees: an overview and their use in medicine. *Journal of Medical Systems*, 26, 445-463.
- Rodriguez-Galiano, V. F., Ghimire, B., Rogan, J., Chica-Olmo, M., & Rigol-Sanchez, J. P. (2012). An assessment of the effectiveness of a random forest classifier for land-cover classification. *ISPRS Journal of Photogrammetry and Remote Sensing*, 67, 93-104.
- Sajid, S. (2018). Prostate cancer dataset. [Online]. Available: [https://www.kaggle.com/sajid-saifi/prostate-cancer](https://www.kaggle.com/sajid-saifi/prostate-cancer)
- Saritas, M. M., & Yasar, A. (2019). Performance analysis of ANN and Naive Bayes classification algorithm for data classification. *International Journal of Intelligent Systems and Applications in Engineering*, 7(2), 88-91.
- Yong, X., & Gao, Y. L. (2023). Improved firefly algorithm for feature selection with the ReliefF-based initialization and the weighted voting mechanism. *Neural Computing and Applications*, 35(1), 275-301.