MNETGIDD: A heuristic-oriented segmentation and deep learning multi-disease detection model for gastrointestinal tracts
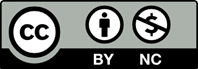
Malignant growth of the gastrointestinal (GI) tract is among the leading causes of death worldwide. Research indicates that almost 40% of people worldwide suffer from long-term digestive issues. According to a study published in the United European Gastroenterology Journal, digestive disorders have increased since 2000. Digestive disorders continue to be a major cause of death, even with a slight decline. The World Health Organization’s Mortality Database reported huge death rates every year due to GI diseases. From that report, the need to accurately detect GI tract malignant in low-cost and error-prone labor must be developed. This work introduces MNET Gastrointestinal Disease Detection (MNETGIDD), which is a complete identification model for multi-gastrointestinal disease discovery from clinical images. MNETGIDD model uses the Gastrolab dataset with endoscopic images, acting as pipelines that are pre-processed and segmented to identify the affected areas. This proposed approach aims to enhance image quality and facilitate accurate segmentation and classification through a pipeline process, initially preprocessing with techniques such as text removal, illumination enhancement, and fuzzy histogram equalization. During segmentation, Otsu segmentation based on Krill-Herd optimization was used to identify the affected area. The MNETGIDD model incorporates the MobileNetV2 architecture, designed for a lightweight classification model working under resource-constrained environments. According to the tests, the MNETGIDD model exhibits high sensitivity and specificity, often outperforming human experts. In terms of accuracy, the model achieved 96.349%, a precision of 96.25 %, and a recall of 97.08%. This deep learning system has the potential to revolutionize gastrointestinal disease diagnostics and screening by automating key steps and improving patient outcomes.
- Al-Adhaileh, M.H., Senan, E.M., Alsaade, F.W., Aldhyani, T.H.H, Alsharif, N., Alqarni, A.A, Uddin, M.I., Alzahrani, M.Y., Alzain, E.D. & Jadhav, M.E. (2021). Deep Learning Algorithms for Detection and Classification of Gastrointestinal Diseases, Complexity, 2021:12, https://doi.org/10.1155/2021/6170416
- Alatab, S., Sepanlou, S.G. & Ikuta, K. (2020) The global, regional, and national burden of inflammatory bowel disease in 195 countries and territories, 1990–2017: a systematic analysis for the Global Burden of Disease Study 2017. The Lancet Gastroenterology and Hepatology. 5(1)17-30
- Cogan, T., Cogan, M. & Tamil, L. (2019). MAPGI: Accurate identification of anatomical landmarks and diseased tissue in gastrointestinal tract using deep learning, Computers in Biology and Medicine, 111, https://doi.org/10.1016/j.compbiomed.2019.103351.
- Ekiri, A.B., Long, M.T. & Hernandez, J.A. (2016). Diagnostic performance and application of a real-time PCR assay for the detection of Salmonella in fecal samples collected from hospitalized horses with or without signs of gastrointestinal tract disease, The Veterinary Journal, 208, 28-32, 1090-0233, https://doi.org/10.1016/j.tvjl.2015.11.011.
- Gammulle, H., Denman, S., Sridharan, S. & Fookes, C. (2020). Two-stream deep feature modelling for automated video endoscopy data analysis. Proceedings of the lecture notes in computer science, 12263,742-751, https://doi.org/10.1007/978-3-030-59716-0_71
- Gandomi, A.H. & Alavi, A.H. (2012). Krill herd: A new bio-inspired optimization algorithm, Communications in Nonlinear Science and Numerical Simulation, 17(12):4831-4845, 1007-5704, https://doi.org/10.1016/j.cnsns.2012.05.010.
- Gastrolab—The Gastrointestinal Site. Available online: http://www.gastrolab.net/
- Govindaprabhu, G.B. & Sumathi, M. (2024a). Ethno medicine of Indigenous Communities: Tamil Traditional Medicinal Plants Leaf detection using Deep Learning Models. Procedia Computer Science. 235(1):1135-1144. https://doi.org/10.1016/j.procs.2024.04.108.
- Govindaprabhu G.B &Sumathi, M. (2024b). Safeguarding Humans from Attacks Using AI-Enabled (DQN) Wild Animal Identification System, International Research Journal of Multidisciplinary Scope, 5(3), pp. 285–302. https://doi.org/10.47857/irjms. 2024.v05i03.0697.
- Gunasekaran, H., Ramalakshmi, K., Swaminathan, D.K., A, J. & Mazzara, M. (2023). GIT-Net: An Ensemble Deep Learning-Based GI Tract Classification of Endoscopic Images. Bioengineering (Basel). 5;10(7):809. https://doi.org/10.3390/bioengineering10070809.
- Jain, S., Seal, A., Ojha, A., Krejcar, O., Bureš, J., Tachecí, I. & Yazidi, A. (2020). Detection of abnormality in wireless capsule endoscopy images using fractal features, Computers in Biology and Medicine, 127, Article 104094, https://doi.org/10.1109/TITB.2003.813794.
- Jain, S., Seal, A., Ojha, A., Yazidi, A., Bures, J., Tacheci, I. & Krejcar, O. (2021). A deep CNN model for anomaly detection and localization in wireless capsule endoscopy images. Computers in Biology and Medicine, 137, 104789, https://doi.org/10.1016/j.media.2021.102007.
- Jha, D., Ali, S., Hicks, S., Thambawita, V., Borgli, H. & P.H. (2021). A comprehensive analysis of classification methods in gastrointestinal endoscopy imaging. Medical Image Analysis, 70, https://doi.org/10.1016/j.media.2021.102007.
- Johnson, K.P., Chen, L., Patel, S. & Yamamoto, T. (2023). Artificial intelligence in gastrointestinal disease diagnosis: A comprehensive meta-analysis. Nature Digital Medicine, 6, 84. https://doi.org/10.1038/s41746-023-00784-2.
- Lonseko, Z.M., Adjei, P.E., Du, W., Luo, C., Hu, D., Zhu L., Gan T. & Rao N. (2021). Gastrointestinal Disease Classification in Endoscopic Images Using Attention-Guided Convolutional Neural Networks. Applied Science, 11. https:// doi.org/10.3390/app112311136.
- Melaku, B.H., Ayodeji, O.S., Belay, E., Abebech, J.B. & Zhongmin, J. (2022). Detection and classification of gastrointestinal disease using convolutional neural network and SVM, BIOMEDICAL ENGINEERING, Cogent Engineering, 9(1).
- Naz, J., Sharif, M., Yasmin, M., Raza, M. & Khan, M.A. (2021). Detection and Classification of Gastrointestinal Diseases using Machine Learning. Current Medical Imaging, 17(4): 479-490.https://doi.org/10.2174/1573405616666200928144626.
- Nguyen, P.T., Le, M.Q., Dao, Q.T., Tran, V.A., Dao, V.H. & Tran, T.H. (2022). Automatic classification of upper gastrointestinal tract diseases from endoscopic images, 11th International Conference on Control, Automation and Information Sciences (ICCAIS), Hanoi, Vietnam. 442-447, https://doi.org/10.1109/ICCAIS56082.2022.9990445.
- Peery, A.F., Crockett, S.D. & Murphy, C.C. (2022). Burden and Cost of Gastrointestinal, Liver, and Pancreatic Diseases in the United States: Update 2021,” Gastroenterology, 162(2), 621–644, https://doi.org/10.1053/j.gastro.2021.10.017.
- Ramamurthy, K., George, T.T., Shah, Y. & Sasidhar, P. (2022). A Novel Multi-Feature Fusion Method for Classification of Gastrointestinal Diseases Using Endoscopy Images. Diagnostics. 12(10):2316. https://doi.org/10.3390/diagnostics12102316.
- Sharib, A., Mariia, D., Noha, G., Sophia, B., Gorkem, B., Alptekin, T., Adrian, K., Amar, H. & Yun, B.G. (2021). Deep learning for detection and segmentation of artefact and disease instances in gastrointestinal endoscopy, Medical Image Analysis, 70, 1361-8415, https://doi.org/10.1016/j.media.2021.102002.
- Sharma, A., Kumar, R. & Garg, P. (2023). Deep learning-based prediction model for diagnosing gastrointestinal diseases using endoscopy images, International Journal of Medical Informatics, 177, 1386-5056, https://doi.org/10.1016/j.ijmedinf.2023.105142.
- Sharmila, V. & Geetha, S. (2022). Detection and Classification of GI-Tract Anomalies from Endoscopic Images Using Deep Learning, IEEE 19th India Council International Conference (INDICON), Kochi. 1-6, https://doi.org/10.1109/INDICON56171.2022.10039766.
- Smith, J.A., Brown, T.L. & Garcia, R.M. (2022). Impact of early detection on survival rates in colorectal cancer: A 10-year retrospective study. Journal of Gastrointestinal Oncology. 37(4), 562-571. https://doi.org/10.1000/jgo.2022.05.023
- Su, Q., Wang, F., Chen, D., Chen, G., Li, C. & Wei, L. (2022). Deep convolutional neural networks with ensemble learning and transfer learning for automated detection of gastrointestinal diseases, Computers in Biology andMedicine, 150, 0010-4825, https://doi.org/10.1016/j.compbiomed.2022.106054.
- Sung, H., Ferlay, J., Siegel, R.L. & Laversanne, M. (2021). Global Cancer Statistics 2020: GLOBOCAN Estimates of Incidence and Mortality Worldwide for 36 Cancers in 185 Countries,CA a Cancer Journal for Clinicians, 71(3), 209–249, https://doi.org/10.3322/caac.21660.
- Theo, V. (2019). “Global burden of 369 diseases and injuries in 204 countries and territories, 1990–2019: a systematic analysis for the Global Burden of Disease Study”, The Lancet, 396: 10258, 1204 –1222.
- Uçan, M., Kaya, B. & Kaya, M. (2022). Multi-Class Gastrointestinal Images Classification Using EfficientNet-B0 CNN Model, 2022 International Conference on Data Analytics for Business and Industry (ICDABI), Sakhir, Bahrain. 1-5, https://doi.org/10.1109/ICDABI56818.2022.10041447.
- Wong, W.N., Wong, Y.K. & Chan, W.H. (2022). Classification of Gastrointestinal Diseases Using Deep Transfer Learning, 2nd International Conference on Intelligent Cybernetics Technology & Applications (ICICyTA),Bandung, Indonesia, 156-161, https://doi.org/10.1109/ICICyTA57421.2022.10038047.
- Yogapriya, J., Chandran, V., Sumithra, M.G., Anitha, P., Jenopaul, P. & Dhas, C.S.G, (2021). Gastrointestinal Tract Disease Classification from Wireless Endoscopy Images Using Pretrained Deep Learning Model, Computational and Mathematical Methods in Medicine, 2021-12, https://doi.org/10.1155/2021/5940433