Predictive analytics: Unveiling the potential of machine learning and deep learning
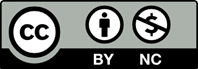
Machine and deep learning methods have gained significant prominence in the healthcare industry, particularly for the prediction of cardiac diseases. The increasing prevalence of heart-related diseases underscores the necessity for proactive and accurate healthcare interventions. Machine learning, a data-driven approach, can play a crucial role in recognizing and addressing cardiovascular risks. To achieve this, researchers have utilized a range of classification techniques, such as Support Vector Machines, Random Forests, and Naive Bayes, to unravel the intricate aspects of heart disease prediction. Additionally, ensemble learning techniques, especially Stacking Technique, is employed to further enhance predictive accuracy. However, the ensemble approach has certain limitations. Therefore, confusion matrices are utilized for thorough evaluation and validation, offering better classifier performance. As research advances, prediction models aim to achieve higher accuracy and generalizability. Insights from confusion matrices can help researchers to make more robust and dependable predictions.Future research will investigate the deep learning models to detect subtle patterns in electrocardiogram data, with the aim of enabling earlier identification of cardiovascular conditions. Additionally, the integration of wearable sensor technologies holds promise for continuous risk monitoring and the development of personalized healthcare interventions. These technological advancements possess the potential to fundamentally transform the field of cardiac care, facilitating earlier disease diagnosis and substantially enhancing patient prognosis. In conclusion,the convergence of machine learning and deep learning models heralds a novel era for precision medicine, where data-driven insights empower stakeholders to tackle formidable challenges with unparalleled effectiveness.
- Agrawal S., Revolutionizing Cardiovascular Health: A Machine Learning Approach for Predictive Analysis and Personalized Intervention in Heart Disease, Int J Res Appl Sci Eng Technol, vol. 12, no. 3, pp. 233–246, Mar. 2024, doi: 10.22214/ijraset.2024.58797.
- Animesh Hazra, Subrata Kumar Mandal, Amit Gupta, Arkomita Mukherjee & Asmita Mukherjee, Heart Disease Diagnosis and Prediction Using Machine Learning and Data Mining Techniques: A Review, Advances in Computational Sciences and Technology, ISSN 0973-6107 Volume 10, Number 7 (2017) pp. 2137-2159
- Bellman, R.E. & Zadeh, L.A. (1970). Decision-making in a fuzzy environment, Management Science, 17(4), 141-164.
- Chaudhary I. & Anwar H., The Impact of Artificial Intelligence on Cardiovascular Disease Diagnosis: A Review, Pakistan Journal of Medical and Health Sciences, vol. 17, no. 11, pp. 8–13, Feb. 2024, doi: 10.53350/pjmhs0202317118.
- Dangare C. S. & Apte S. S., Improved Study of Heart Disease Prediction System using Data Mining Classification Techniques, Int J Comput Appl, vol. 47, no. 10, pp. 44–48, Jun. 2012, doi: 10.5120/7228-0076.
- Faldu P., Menaria S., Patel T., Mt.-C. Student, and A. Professor, “Issue 12 www.jetir.org (ISSN-2349-5162),” 2019. [Online]. Available: www.jetir.org
- Hlaudi Daniel Masethe etal., Prediction of Heart Disease using Classification Algorithms, Proceedings of the World Congress on Engineering and Computer Science [WCECS], Volume: II, ISBN: 978-988-37253-7-4 ISSN: 2078-0958 (Print), ISSN: 2078-0966 (Online) (2014).
- Hsu, W.L. (2008). Decision Model of Product Design, Ph.D. Dissertation of Folks University, Taiwan. (in Chinese).
- Maganathan T., SenthilkumarS., & Balakrishnan V., Machine Learning and Data Analytics for Environmental Science: A Review, Prospects and Challenges, in IOP Conference Series: Materials Science and Engineering, IOP Publishing Ltd, Nov. 2020. doi: 10.1088/1757-899X/955/1/012107.
- Mandal S., “Heart Disease Diagnosis and Prediction Using Machine Learning and Data Mining Techniques: A Review,” 2017. [Online]. Available: http://www.ripublication.com.
- Naseer A., Muhammad, Khan M., Arif F., Iqbal W., and Ahmad A., “An Improved Hybrid Model for Cardiovascular Disease Detection Using Machine Learning in IoT.
- Polaraju K., Durga Prasad D., and Tech Scholar M., Prediction of Heart Disease using Multiple Linear Regression Model, 2017. [Online]. Available: www.ijedr.org.
- Shakkeera L. & Kavitha P.. (2024). Predictive Modelling for Medical Image Analysis Using Deep Learning Techniques. International Journal on Recent and Innovation Trends in Computing and Communication, 12(1), 291–298.from https://ijritcc.org/index.php/ijritcc/article/view/10327.
- Sharmila S. et al., Analysis of Heart Disease Prediction using Data Mining Techniques, International Journal of Advanced Networking & Applications (IJANA), Volume: 08, Issue: 05, Pages: 93-95 (2017).
- Srinivasa Rao G. & Muneeswari G., A Review: Machine Learning and Data Mining Approaches for Cardiovascular Disease Diagnosis and Prediction, EAI Endorsed Trans Pervasive Health Technol, vol. 10, Mar. 2024, doi: 10.4108/eetpht.10.5411.
- Theresa Princy R, Human Heart Disease Prediction System using Data Mining Techniques, International Conference on Circuit, Power and Computing Technologies [ICCPCT], IEEE (2016).
- Thansekhar M. R. & Balaji N., Heart Disease Diagnosis using Predictive DataMining. [Online]. Available: http://www.cs.waikato.ac.nz/ml/weka.