Structure learning of Bayesian networks using sparrow optimization algorithm
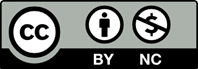
Bayesian networks are powerful analytical models in machine learning, used to represent probabilistic relationships among variables and create learning structures. These networks are made up of parameters that show conditional probabilities and a structure that shows how random variables interact with each other. The structure is shown by a directed acyclic graph. Despite the NP-hard nature of learning Bayesian network structures, there has been significant progress in improving the accuracy of approximation solutions. The main focus is on score-based search strategies, which make use of functions to evaluate network models and identify structures with high scores. This study is significantly focused on structure learning Bayesian networks using the Bayesian Dirichlet equivalent uniform scoring function and metaheuristic search strategies. To this end, this paper presents the sparrow optimization algorithm (SOA), a new metaheuristic algorithm derived from the foraging behavior of sparrows. SOA performs a concurrent optimization in the solution space by simultaneously performing a local and global search that leads to the discovery of near-optimal structures. The results from our experiments on several benchmark datasets show that SOA yields overall better performance than SA and greedy search algorithms. In particular, it is claimed that by applying the proposed approach of SOA, the convergence speed is significantly higher compared with the existing ones; F1 score is 0.35 and 0.05 for the Hamming distance with better results. Given these results, signed operators prove to be very efficient in SOA’s Bayesian network structure learning as a concept, especially for real-world use.
- Askari, M.B.A., & Ahsaee, M.G. (2018). Bayesian Network Structure Learning Based on Cuckoo Search Algorithm. In: 6th Iranian Joint Congress on Fuzzy and Intelligent Systems (CFIS). Kerman, Iran.
- Campos, L.M. (2006). A scoring function for learning Bayesian networks based on mutual information and conditional independence tests. Journal of Machine Learning Research, 7, 2149–2187.
- Cooper, G.F., & Herskovits, E. (1992). A Bayesian method for the induction of probabilistic networks from data. Machine Learning, 9, 309–347. https://doi.org/10.1007/BF00994110
- Cowie, J., Oteniya, L., & Coles, R. (2007). Particle Swarm Optimisation for Learning Bayesian Networks. Engineering and Physical Sciences Research Council, United Kingdom.
- Dekker, D. (2009). Hunting Tactics of Peregrines and Other Falcons. Wageningen University, Wageningen, The Netherlands.
- Djan-Sampson, P.O., & Sahin, F. (2004). Structural Learning of Bayesian Networks from Complete Data Using the Scatter Search Documents. In: IEEE International Conference on Systems, Man and Cybernetics. IEEE, The Hague, Netherlands. https://doi.org/10.1109/ICSMC.2004.1400904
- Fan, X., Yuan, C., & Malone, B. (2014). Tightening Bounds for Bayesian Network Structure Learning. Association for the Advancement of Artificial Intelligence, Washington, DC.
- Fast, A.S. (2010). Learning the Structure of Bayesian Networks with Constraint Satisfaction. Ph.D. Thesis, Department of Computer Science, University of Massachusetts.
- Fortier, N., Sheppard, J., & Pillai, K.G. (2013). Bayesian Abductive Inference Using Overlapping Swarm Intelligence. In: IEEE Symposium on Swarm Intelligence. IEEE, Singapore. https://doi.org/10.1109/SIS.2013.6615188
- Gadekallu, T.R., & Khare, N. (2017). Cuckoo search optimized reduction and fuzzy logic classifier for heart disease and diabetes prediction. International Journal of Fuzzy System Applications, 6, 25–42. https://doi.org/10.4018/IJFSA.2017040102
- Gandomi, A.H., Yang, X.S., Talatahari, S., & Alavi, A.H. (2013). Metaheuristic Applications in Structures and Infrastructures. Elsevier, USA.
- He, C., & Gao, X. (2018). Structure Learning of Bayesian Networks Based on the LARS-MMPC Ordering Search Method. In: 2018 37th Chinese Control Conference (CCC). IEEE, Wuhan, China.
- Heckerman, D., Geiger, D., & Chickering, D.M. (1995). Learning Bayesian networks: The combination of knowledge and statistical data. Machine Learning, 30, 197–243. https://doi.org/10.1023/A:1022623210503
- Hedenström, A., Rosén, M., Åkesson, S., & Spina, F. (1999). Flight performance during hunting excursions in Eleonora’s Falcon Falco Eleonorae. Journal of Experimental Biology, 202, 2029–2039. https://doi.org/10.1242/jeb.202.15.2029
- Hesar, A.S. (2013). Structure learning of Bayesian belief networks using simulated annealing algorithm. Middle-East Journal of Scientific Research, 18, 1343–1348. https://doi.org/10.5829/idosi.mejsr.2013.18.9.12375
- Ji, J., Wei, H., & Liu, C. (2012). An Artificial Bee Colony Algorithm for Learning Bayesian Networks. Springer-Verlag, Berlin, Heidelberg.
- Kareem, S.W., & Okur, M.C. (2019). Bayesian network structure learning based on pigeon-inspired optimization. International Journal of Advanced Trends in Computer Science and Engineering, 8(1.2), 131–137. https://doi.org/10.30534/ijatcse/2019/2281.22019
- Kareem, S.W., & Okur, M.C. (2018). Bayesian Network Structure Learning Using Hybrid Bee Optimization and Greedy Search. In: 3rd International Mediterranean Science and Engineering Congress. Adana, Turkey.
- Khanteymoori, A., Olyaee, M.H., Abbaszadeh, O., & Valian, M. (2018). A novel method for Bayesian networks structure learning based on breeding swarm algorithm. Soft Computing, 9, 1–12. https://doi.org/10.1007/s00500-017-2557-z
- Larrañaga, P., & Poza, M. (1996). Structure Learning of Bayesian Networks by Genetic Algorithms. Springer-Verlag, Berlin Heidelberg.
- Li, J., & Chen, J. (2014). A hybrid optimization algorithm for Bayesian network structure learning based on database. Journal of Computers, 9.
- Li, S., & Wang, B. (2017). A Method for Hybrid Bayesian Network Structure Learning from Massive Data Using MapReduce. In: 2017 IEEE 3rd International Conference on Big Data Security on Cloud (Bigdatasecurity), IEEE International Conference on High Performance and Smart Computing (HPSC), and IEEE International Conference on Intelligent Data and Security (IDS). IEEE, Beijing, China. https://doi.org/10.1109/BigDataSecurity.2017.42
- Margaritis, D. (2003). Learning Bayesian Network Model Structure from Data. (Technical Report CMU). Carnegie-Mellon University, Pittsburgh, PA.
- Mirjalili, S., Mirjalili, S.M., & Lewis, A. (2014). A grey wolf optimizer. Advances in Engineering Software, 69, 46–61. https://doi.org/10.1016/j.advengsoft.2013.12.007
- Nagarajan, R., Scutari, M., & Lèbre, S. (2013). Bayesian Networks in R with Applications in Systems Biology. Springer, New York.
- Orphanou, K., Thierens, D., & Bosman, P.A.N. (2018). Learning Bayesian Network Structures with GOMEA. In: GECCO 2018 - Proceedings of the 2018 Genetic and Evolutionary Computation Conference. Kyoto, Japan. https://doi.org/10.1145/3205455.3205502
- Rahier, T., Marie, S., Girard, S., & Forbes, F. (2019). Fast Bayesian network structure learning using quasi-determinism screening. HAL, 2, 14–24.
- Reddy, G.T., & Khare, N. (2016). FFBAT-optimized rule-based fuzzy logic classifier for diabetes. International Journal of Engineering Research in Africa, 24, 137–152. https://doi.org/10.4028/www.scientific.net/JERA.24.137
- Salama, K.M., & Freitas, A.A. (2012). ABC-Miner: An ant-based Bayesian classification algorithm. Swarm Intelligence. In: ANTS 2012. Lecture Notes in Computer Science. Springer, Berlin, Heidelberg. https://doi.org/10.1007/978-3-642-32650-9_2
- Segundo, E.H.V., Mariani, V.C., & Coelho, L.S. (2019). Design of heat exchangers using Falcon Optimization Algorithm. Applied Thermal Engineering, 156, 119–144. https://doi.org/10.1016/j.applthermaleng.2019.04.038
- Sencer, S., Oztemel, E., Taskin, H., & Torkul, O. (2013). Bayesian Structural Learning with Minimum Spanning Tree Algorithm. In: The World Congress in Computer Science, Computer Engineering, and Applied Computing.
- Tucker, V.A. (1998). Gliding flight: Speed and acceleration of ideal falcons during diving and pull out. Journal of Experimental Biology, 201, 403–414. https://doi.org/10.1242/jeb.201.3.403
- Tucker, V.A. (2000). Gliding flight: Drag and torque of a hawk and a falcon with straight and turned heads, and a lower value for the parasite drag coefficient. Journal of Experimental Biology, 203, 3733–3744. https://doi.org/10.1242/jeb.203.24.3733
- Wang, J., & Liu, S. (2018). Novel binary encoding water cycle algorithm for solving Bayesian network structures learning problem. Knowledge-Based Systems, 150, 95–110. https://doi.org/10.1016/j.knosys.2018.03.007
- Yang, C., Ji, J., Liu, J., Liu, J., & Yin, B. (2016). Structural learning of Bayesian networks by bacterial foraging optimization. International Journal of Approximate Reasoning, 69, 147–167. https://doi.org/10.1016/j.ijar.2015.11.003
- Yuan, C., Malone, B., & Wu, X. (2011). Learning optimal Bayesian networks using A* search. In: Proceedings of the 22nd International Joint Conference on Artificial Intelligence (IJCAI). Barcelona.
- Zhang, S.Z., & Liu, L. (2008). MCMC Samples Selecting for Online Bayesian Network Structure Learning. In: International Conference on Machine Learning and Cybernetics. IEEE, Kunming.https://doi.org/10.1109/ICMLC.2008.4620690